
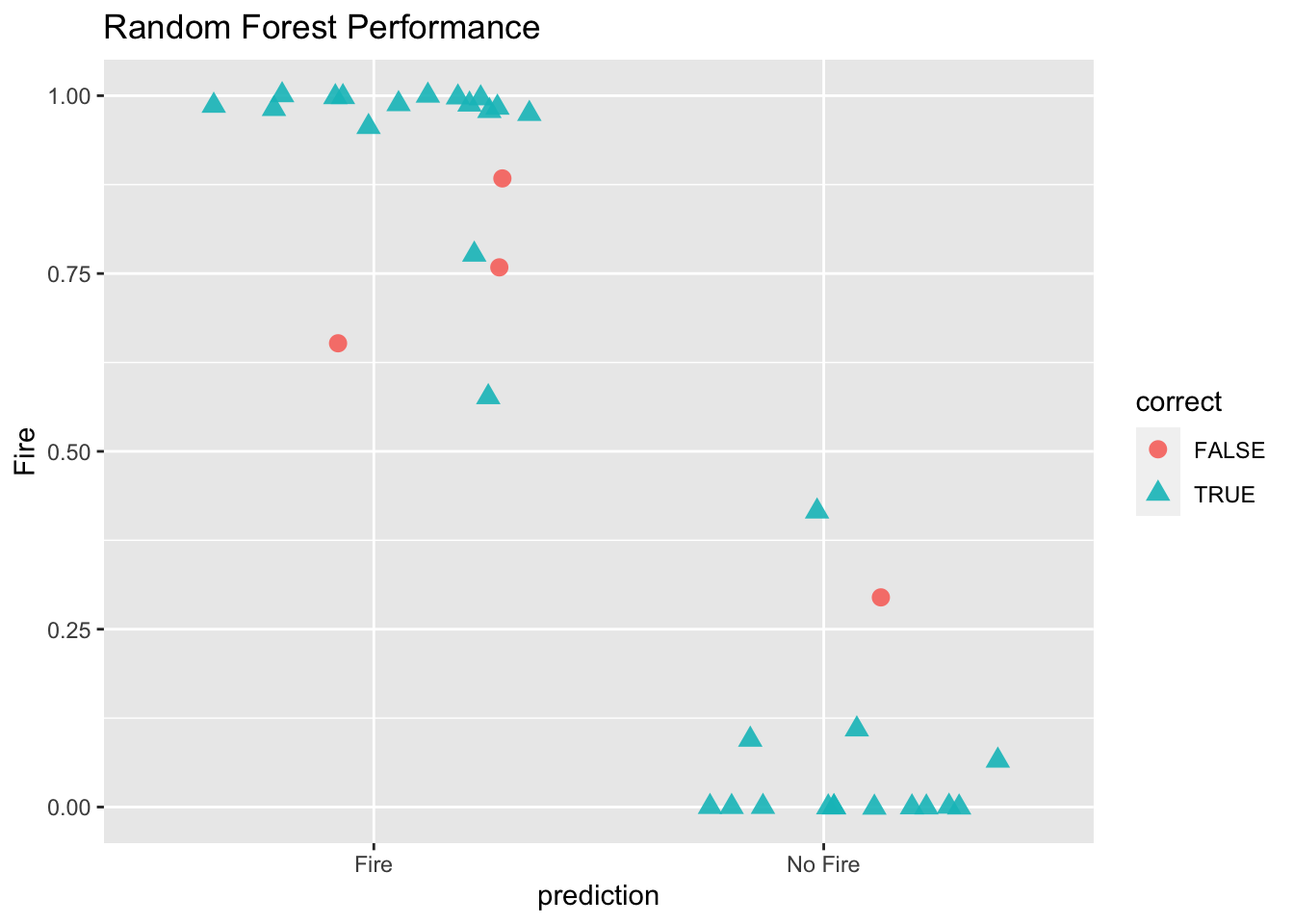

You get a lower RMSE because the data your are predicting is present in the model you have constructed, whereas it was not the case when train evaluated the performance of the models.
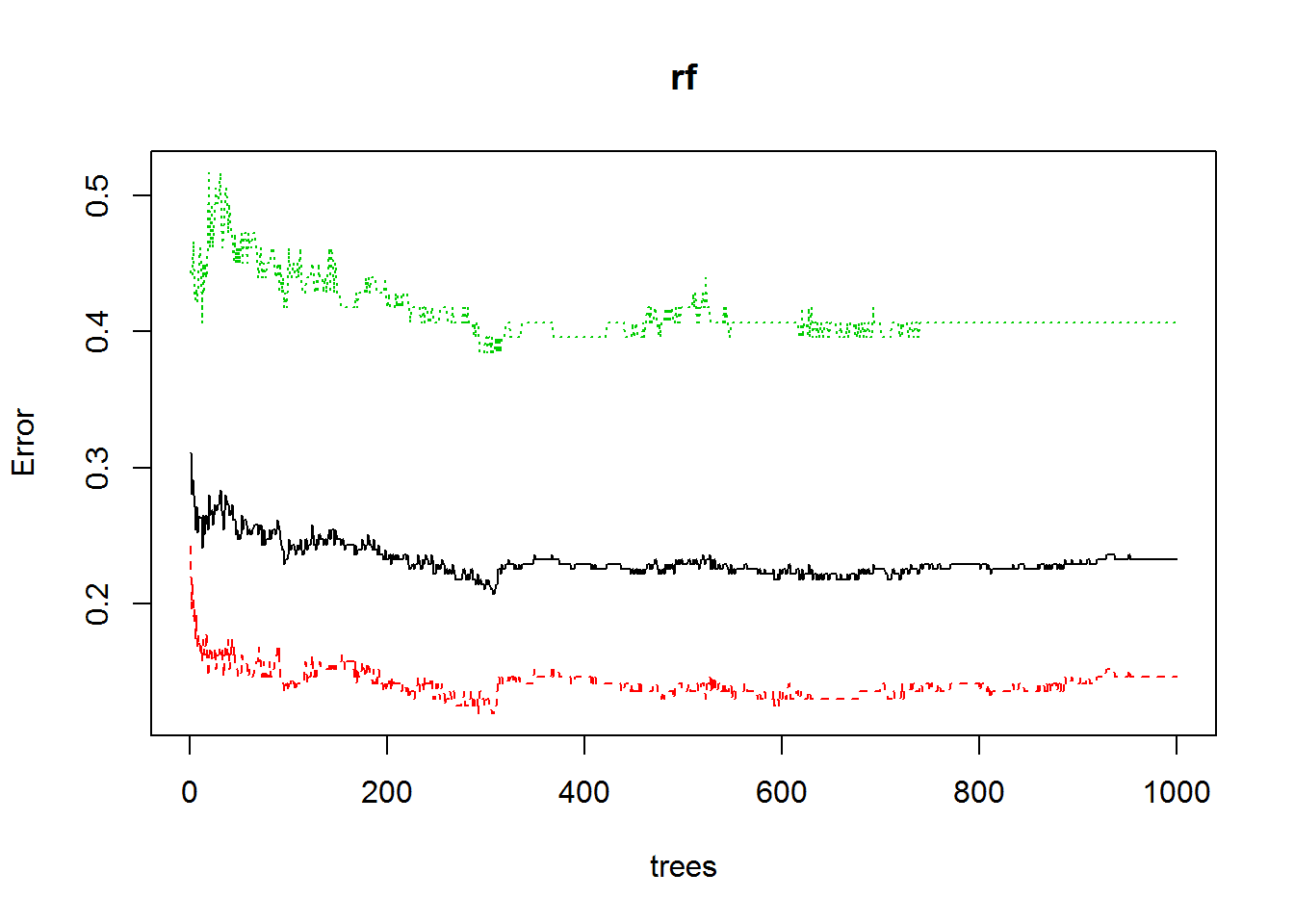
So when your are predicting your traindat with the final rf model and calculating the resulting RMSE, you are not comparing the same things. Obviously, the final model uses all your data with the optimal calculated parameters - in your case, mtry = 3. So the RMSE displayed in rf is the RMSE calculated on the sub-testing sets, based on the model built with the sub-validation sets (hence, distinct datasets for training and testing). What did I do wrong? Is ain the right way to do predictions for random forests?Īs per documentation of train and trainControl, there is a sampling / cross-validation process which separates your training set into a "sub-training" set and a "sub-validation" set to build the model.ĭefault value for separation is 0.75, which means that at each iteration of the cross-validation, 75% of your values are used to build the sub-training set and the remaining 25% (sub-testing set) are tested. Random Forest Regression using Caret Raw metabolomics.R This file contains bidirectional Unicode text that may be interpreted or compiled differently than what. If we have only two values, the importance of the variable as a predictor can be represented as one value. I went on to check for this RMSEa and tried to calculate it by hand: > sqrt(mean((ain(rf, newdata = traindat, type = "raw") - traindat$Sepal.Length)^2)) Random Forest: varImp.randomForest and varImp.RandomForest are wrappers around the importance functions from the randomForest and party packages, respectively. This tells me that the parameter mtry = 3 performed best on the training set with a RMSE of 0.33. The final value used for the model was mtry = 3. RMSE was used to select the optimal model using the smallest value. Resampling results across tuning parameters: Resampling: Cross-Validated (10 fold, repeated 3 times)
#CARET RANDOM FOREST HOW TO#
I recently got confused on how to do correct predictions for random forrests.
